The advancement of generative AI, particularly multimodal models, is set to revolutionize e-commerce, lifting it from a basic transactional process to an experience akin to human-level engagement. In time, users will find themselves in a virtual shopping world where the system both interprets and produces content in forms such as text, images, videos, and sound, mirroring human abilities. The potential of these advanced e-commerce systems will be enormous, constrained only by the limits of human imagination and the speed of technological progress.
In the upcoming sections, I will first delve into three key areas where these advancements are manifesting: 1) the evolution of e-commerce interactions to more human-like experiences in understanding intent and offering clear responses; 2) the deep customization of e-commerce with tailored interfaces; and 3) the opportunities of AI empowered content generation. Afterward, I will examine the social and commercial implications as AI gets deep insights of human needs.
Human-Like Interactions
Traditional e-commerce platforms offer limited ways for users to interact with the system. Users must express their intent clearly through a bag of keywords, which is sometimes a cognitive burden. This issue, along with the system’s opaque logic in product recommendations and rankings, often leaves users puzzled about why certain items are suggested or prioritized, leading to a disappointing customer experience that could erode their trust.
Specifically, the challenge of interpretability in modern e-commerce systems mainly stems from two issues: 1) the systems’ lack of essential knowledge needed for clear explanations, and 2) their reliance on ‘non-interpretable’ algorithms, which use “implicit” representations (like embeddings) of entities such as products and customers, obscuring the rationale behind their decisions.
LLMs are beginning to break down these barriers. For instance, Google’s Generative AI experiment now responds to queries like “mother’s day gifts” in more understandable ways, with intuitive gift categories and even offers to create personalized gifts featuring a mom’s name, initials, or favorite photos.

The adoption of dialogue and voice interfaces in e-commerce is an expected evolution. For over twenty years, users have been trained to use keyword-based queries on search engines, but this was more a limitation of the information retrieval systems’ capacity to handle natural language than a preference. As shown in Fig. 5, there’s a growing trend of users turning to natural language for expressing their intentions on Google. This trend is likely to extend to e-commerce platforms too, where users seek not only products that meet their needs but also insights into how these products function and their advantages.

Today, LLM-powered chatbots are already enhancing customer-system interactions. In 2023, several e-commerce platforms such as Instacart, Shopify, and Mercari introduced chatbots. Fig. 6 shows an example of the Mercari chatbot, engaged in dialogue with the customer, asking for preferences to make recommendations.

Conversation technology is still in its infancy, as highlighted in a New York Times report on chatbot experiences during the 2023 holiday season. However, generative AI has the potential to rapidly evolve these interactions to be more human-like. Future iterations could include features like sentiment analysis to gauge customer mood and adjust responses accordingly. Overall, advancements in understanding context, sentiment, and user behavior can make these chatbots more intuitive, responsive, and personalized. As these technologies mature, they will help close the gap between digital and human communication in e-commerce.
Tailored Interfaces
Transitioning from keyword searches to human language queries and dialogues is a significant step forward in e-commerce, yet there’s a need to go beyond mere text.
Online furniture retailers recognize that customers may find it hard to articulate their specific furniture preferences, such as the exact style of a sofa. To tackle this, furniture retailers have developed a mechanism where customers are shown a variety of sofa styles based on their initial search. As customers select the options that most appeal to them, the system gradually refines its understanding of their preferences. This approach, while indirect, helps the system to implicitly zero in on the customer’s ideal furniture style through their choices, without them needing to explicitly describe what they’re looking for.
Generative AI is taking this a step further by making the communication process more explicit, eliminating guesswork. A notable example is in fashion retail, where virtual try-on technologies, like the ‘Outfit Anyone’ platform (Fig. 7), are poised to change how consumers interact with clothing online. Utilizing a two-stream conditional diffusion model, ‘Outfit Anyone’ successfully tackles the challenge of creating realistic virtual garments that fit different body shapes and poses, offering a more inclusive and creative fashion experience.
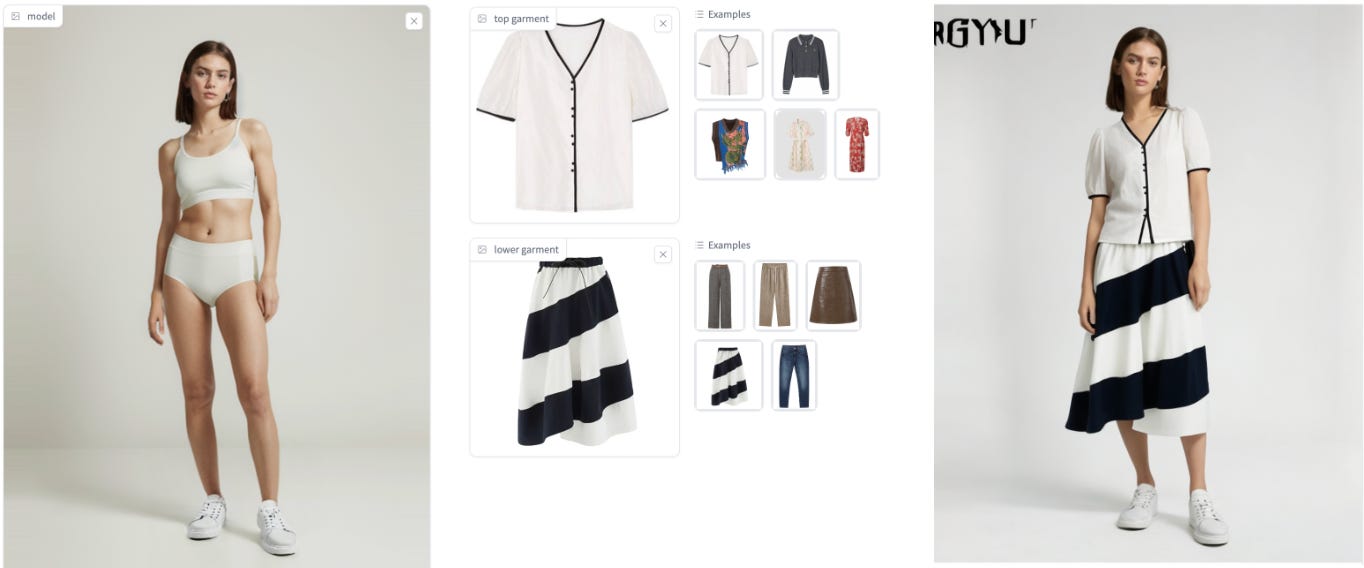
While the fashion industry’s scale justifies the creation of bespoke AI models, crafting such tailored solutions for the entire spectrum of products and every conceivable user intent is not feasible.
Google’s recent showcase of the Gemini generative AI model highlights a ‘no-code’ method for personalizing digital experiences. As shown in Fig. 8, when a user requests “birthday party ideas for my daughter,” Gemini engages in an interactive dialogue to learn the child’s interests. Upon discovering her love for animals and the outdoors, it then crafts a bespoke interface that does more than just enumerate suggestions; it visually showcases an array of animal-themed party options, reflecting the child’s specific interests.

How do we progress from here to a future where online shopping could rival the satisfaction of in-person experiences? It may hinge on generative AI’s capability to acquire and process sensory data.
Today, e-commerce faces a notable disadvantage compared to traditional brick-and-mortar stores: the absence of sensory engagement. Online shoppers are unable to experience the tangible aspects of shopping — feeling the texture of a product, the instant trial of items, from clothes fitting to tech gadget testing, and absorbing the unique atmosphere of a physical store. This lack of sensory interaction creates a noticeable gap in the overall digital shopping experience.
Generative AI has the potential to revolutionize online shopping by bridging this sensory divide. It could enable highly immersive virtual experiences where customers can almost ‘feel’ the products through advanced visualization technologies. Personalized, AI-driven recommendations are set to become more intuitive, understanding customer preferences in a much deeper and more human-like manner. Detailed visual presentations and interactive interfaces will allow customers to explore products in ways that closely mimic the in-store experience.
Content is King
In his 1996 essay, “Content is King,” Bill Gates foresaw the pivotal role of content in the burgeoning digital landscape, particularly in marketing and online communication. For instance, online social networks and various businesses have relied on UGC (user-generated content) for their content needs, and platforms such as Goodreads thrived on book lists created and populated by users.
Bill Gates’ insight, articulated during the internet’s rapid expansion, is more relevant than ever in the era of generative AI. Almost certainly, content creation will undergo a dramatic shift, as generative AI enables the production of vivid, engaging, and customized content at an unprecedented scale and efficiency, significantly lowering costs in the process.
For example, LLMs can produce book lists on highly specialized topics (Fig. 9 shows a book list generated by GPT-4 on the topic of “sci-fi with romantic elements”). While I am not suggesting yet that these AI-generated lists match the insight of those created by human users on Goodreads, it’s worth noting that generative AI is able to produce specialized content that caters to niche user interests.
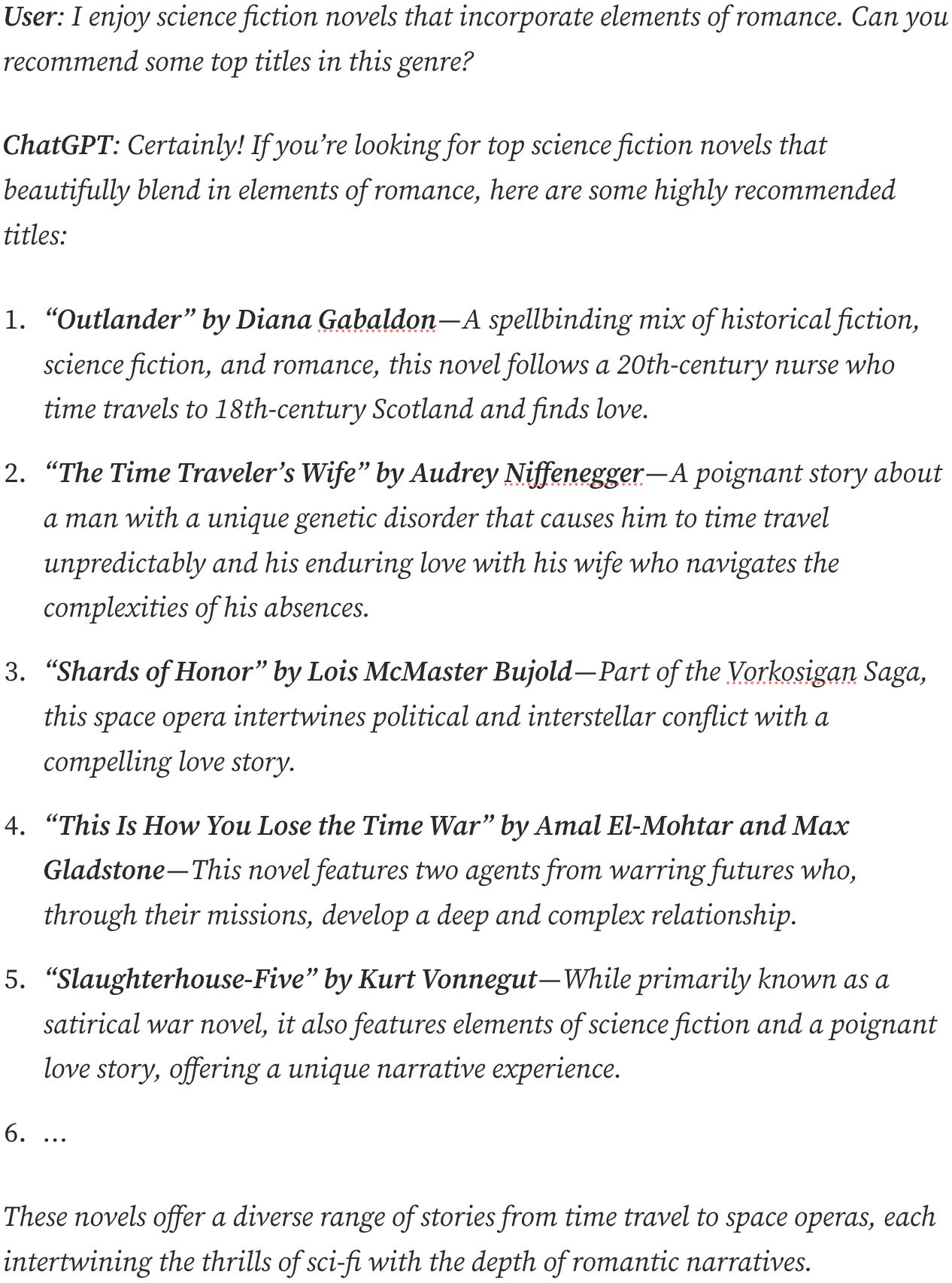
The innovation also doesn’t stop at text. Generative AI can create content in images and videos. This is evident on platforms like Instacart, where AI dynamically creates recipes catered to very specific customer requests, complete with detailed instructions and relevant visuals (see Fig. 10). For example, LLMs can generate recipes based on the ingredients in a customer’s shopping cart or fridge. The combination of customized content with its visual presentation significantly elevates the user experience, showcasing how AI is transforming content generation to become more customizable and interactive.
Besides creating new content, generative AI can also add value to existing content. Consider the multitude of food, recipe, and cooking videos on platforms like YouTube and TikTok. Generative AI can enhance these videos by linking them directly to the ingredients featured. This not only provides viewers with the convenience of purchasing these ingredients with a single click but also opens up new avenues for monetizing content for creators and platforms alike.

Content creation opens up new frontiers beyond improving existing products. It facilitates the creation of new products that integrate AI’s imaginative concepts with real-world materials from e-commerce platforms. Put simply, this approach uses existing products as the “ingredients” to create novel “recipes” and “dishes.”
For example, Material Bank is an online platform that offers an extensive range of free samples of architecture, construction, and design materials. This platform is a boon for interior designers, architects, and construction project managers, providing them with a playground to test and evolve new concepts (Fig. 11). This is comparable to how an inventive chef might view a grocery store — a repository of possibilities for culinary creations. In this evolving scenario, generative AI could act as an inventive design partner, crafting bespoke design solutions and conceptual frameworks from the diverse materials available, thus significantly advancing and refining the creative workflow.

Implications of Deep Personalization
Understanding customer needs is essential for online businesses to provide tailored services. Present personalization methods fall short, but we’re on the cusp of a technological breakthrough that could potentially enable future systems to know customers better than they know themselves. However, these advances come with ethical considerations, especially in e-commerce, where the pursuit of profit might take precedence over user interests.
Current Status
For many years, e-commerce platforms have maintained detailed user profiles, tracked customers’ browsing patterns and purchasing histories, and used the data to make personalized recommendations. How successful has it been?
Elle Hunt’s recent Guardian article offers a critical view of the current personalization practice. Hunt notes, “Every tech company from Monzo to my bank is crunching my data. All the results tell us is how dull it is to reduce human experience to numbers.” This statement highlights a major limitation of today’s personalization technologies.
While companies like Spotify are adept at aggregating quantitative data, they fail to capture the qualitative, emotional aspects of customer experiences. Indeed, reducing complex human activities to stark statistics strips away the nuances that give human experiences meaning. More specifically, simply tracking the number of hours spent listening to Taylor Swift, or the frequency a particular dish is ordered through DoorDash, doesn’t reveal the motivations or feelings behind these actions.
The Rise of Technology
However, we might be surprised just how soon multimodal and generative AI technologies could revolutionize the field of personalization. A digital personal assistant, fueled by these advanced technologies, might have the potential to elevate personalization to new, unprecedented heights.
Instead of tracking basic statistics, the personal assistant, equipped with multimodal generative AI technologies, would have the capability to process a variety of sensory inputs, including visual and auditory cues. It’s easy to envision an assistant capable of assessing a customer’s reactions through facial recognition and tone analysis during virtual try-ons and interactive product consultations, thereby obtaining a deep, empathetic understanding of the individual’s preferences and needs.
In contrast to existing personalization methods that are confined to singular platforms and only analyze user behavior in isolated contexts, this personal assistant has the potential to track an individual’s activities across a multitude of platforms. By being a constant in the individual’s life, it will gain a nuanced understanding of the individual’s actions and motivations, achieving a level of insight that traditional data analysis methods cannot match. It might even come to know the individual more intimately than they know themselves.
With its profound understanding, such a personal assistant could theoretically help the individual in engaging with business platforms, in a more clear and effective way than the individual could alone. This holistic approach to personalization is poised to create a more intuitive and emotionally engaging shopping experience.
A Word of Caution: AI, Ethics, and Profit
While contemplating a personal assistant capable of understanding a customer’s sentiment and emotion, I can’t help thinking about lifelogging. Lifelogging refers to a comprehensive recording of a person’s daily life, often aided by wearable technology or mobile devices. This practice has evolved significantly, with projects like DARPA LifeLog contributing to its development, and contemporary apps like Foursquare and Swarm allowing users to document their lives on their platforms. In 2022, Jennifer Egan, an award winning author, explored a related concept in her novel “The Candy House.” She imagined a world where technology has advanced to the point that individuals can upload their memories to a shared database. The novel suggests a kind of collective consciousness, where the boundaries between personal and shared experiences become blurred.

Feeding lifelogging data to multimodal and generative AI technologies, which are capable of analyzing data in various forms (text, images, videos, sensor data), builders can gain a deeper, more holistic understanding of an individual’s habits, preferences, and needs. We will not only be able to predict the individual’s future behaviors, but also act as a proxy of the individual, interacting with the world on her behalf.
Yet, such advancements in personalization and AI’s enhanced understanding of individuals raise significant ethical issues. This situation echoes the ethical concerns similar to those confronting social networks, which have been criticized for manipulating users’ emotions and fostering addictive behaviors to enhance user engagement and profitability. As businesses gain their power to understand their customers, they may use the power not just to predict but to change customers’ behavior in order to boost sales and ad revenue. As playwright Ayad Akhta pointed out in his essay titled The Singularity is Here, “Our affinities are increasingly no longer our own, but rather are selected for us for the purpose of automated economic gain.”
Therefore, as e-commerce platforms harness the power of generative AI to better understand their customers, there’s an imperative to balance commercial goals with ethical considerations. The potential to use these advancements for positive outcomes, such as promoting health, personal growth, and societal benefits, should be weighed against the commercial opportunities they present, ensuring a responsible and human-centric approach to technology application.